Mission
Training supervised surface anomaly detection models requires obtaining a large number anomalous and anomaly-free examples. While anomaly-free examples are often abundant, anomalous examples are rare and their visual properties may vary significantly, making the acquisition of a representative training set a difficult task. Visual anomaly detection methods are trained on anomaly-free examples only and focus on learning a visual model of the anomaly free appearance and detect deviations from the learned model as anomalies.
Our research focuses on the development of visual anomaly detection methods with an emphasis on the detection and localization of surface defects.
Projects
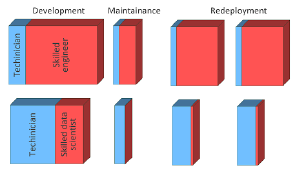
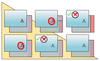