Object counting
We are designing novel deep architectures for object counting under different training regimes: (i) counters based on trainable category-specific detectors and (ii) counters that do not require large training datasets but rely on using only few or no exemplars. The challenges are diverse scales of objects, densely poulated regions and appearance diversity.
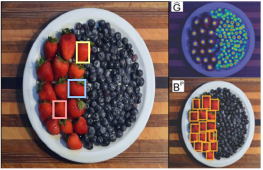
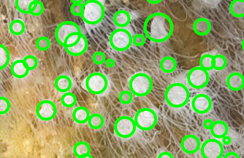