Collaborating partners
- University of Ljubljana, Faculty of Computer and Information Science
- UL, Faculty of Electrical Engineering
Funding
- ARRS (J2-9433)
Project overview
Obtaining a large amount of visual data has become a trivial task in today’s technological world. However, making use of this enormous amount of data poses a huge challenge. Computer vision and machine learning, in particular deep learning, offer answers to these requirements, however most of the approaches proposed rely on labelled training data; they therefore still require a significant human effort for labelling the required amounts of data, which is very costly, tedious and sometimes error-prone or even impossible. In this project we will address this issue for a particular computer vision task of anomaly detection in images. Our aim is to go beyond the traditional supervised learning, where all anomalies on all training images have to be adequately labelled. The objective of the proposed project is to develop novel deep learning methods for modelling complex consistency and detecting inconsistencies in visual data using training images annotated with different levels of accuracy. We will validate the developed methods in three related but different problem domains; visual inspection, remote sensing, and visual surveillance.
The expected contributions of the project are therefore:
- weakly-supervised method for detection and segmentation of anomalies in visual data,
- semi-supervised and self-supervised methods for anomaly detection,
- methods for deep generative modelling of visual consistency for anomaly detection,
- application of anomaly detection methods to visual inspection, remote sensing, and visual surveillance problem domains.
Workpackages: The work programme will be divided into seven work packages. Research will be conducted in the first five work packages that will address the following objectives of the project:
- Development of (weakly) supervised approaches for anomaly detection (WP1).
- Development of novel semi- and self-supervised learning of data consistency for anomaly detection (WP2).
- Development of deep generative compositional models for anomaly detection (WP3).
- Guiding the learning process by considering the cognitive relevance of the learned models (WP4).
- Adaptation of developed data consistency learning for anomaly detection to three problem domains: (i) visual inspection, (ii) remote sensing, and (iii) visual surveillance (WP5).
- The remaining two work packages relate to the dissemination of the results (WP6) and project management (WP7).
Project phases:
- Year 1: Activities on work packages WP1, WP2, WP4, WP5, WP6, WP7
- Year 2: Activities on work packages WP2, WP3, WP4, WP5, WP6, WP7
- Year 3: Activities on work packages WP3, WP4, WP5, WP6, WP7
Results
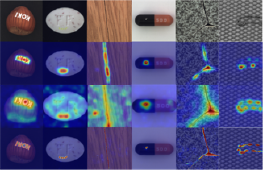
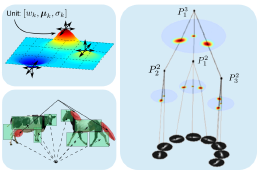
Publications
Financer
ARRS, Slovenian Research Agency